Benchmarks for Machine Learned Material Models
Scope
The project is part of AISA Research, the Phd project branch of the AI Software Academy. In AISA Research, interdisciplinary research projects around the topic of AI are funded. Applications (X), software development (SE) and their didactics are linked with AI to the following: AI+SE+X.
Goals
The number of machine learning methods for the generation of physics-aware constitutive models resulting from in silico or experimental data is steadily increasing. Unfortunately, the ability to compare these methods in a rigorous way is still far from standardized. In order to arrive at a better methodology for comparing—in a quantitative way—competing methods we anticipate the generation of workflows for the automatic execution of simulation, learning and inference, for the automatic scoring and for the analysis of the benchmark results using dedicated visualization and user interfaces. We also plan on creating a knowledge graph for models and data in order to enable researchers to discover suitable methods for their problems at low effort.
Benchmarks for ML Material Models
Funding information and acknowledgement
AISA is funded by the state of Baden-Württemberg through Cyber Valley as a project from the “Zukunftsland BW – Stärker aus der Krise” package of measures. As part of this package, the state government is making targeted investments in important fields of the future, thereby sustainably strengthening the state as a location for business and innovation.
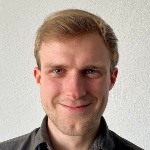
Julius Herb
M. Sc.Doctoral Researcher
[Photo: Julius Herb]
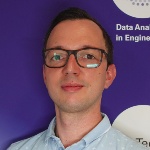
Felix Fritzen
Prof. Dr.-Ing. Dipl.-Math. techn.Chair Holder
[Photo: Felix Fritzen]
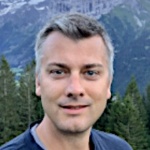
Prof. Dr. Mathias Niepert
- Further information
- Write e-mail
- Machine Learning for Simulation Science