Direct access to
- News
News of the Heisenberg Group for Data Analytics in Engineering - Data-integrated research
reduced order modeling, data-driven modeling and simulation, machine learning, data-integrated multi-scale simulations - Team
All members of the Heisenberg Group for Data Analytics in Engineering
Welcome
Data is our passion
Generating and processing data from simulations and for simulations is our passion. Navigate to research to find out more about our scientific objectives.
Interdisciplinary teaching and student research projects
Our activities comprise dedicated courses for model order reduction and data processing, seminars and colloquia. We are also advertising a variety of student research projects. For more information please visit the overarching institute teaching page.
News and ongoing activities
October 2024
September 2024
August 2024
July 2024
May 2024
October 2023
July 2022
September 2022
March 2023
October 2022
January 2022
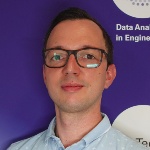
Felix Fritzen
Prof. Dr.-Ing. Dipl.-Math. techn.Head of Department
[Image: Felix Fritzen]